Insights
- The capital markets industry faces many industry and technological headwinds.
- This necessitates the use of generative AI. Generative AI use cases in capital markets can be divided in four areas — building new capabilities, improving operations, fostering innovation, and enabling transformation.
- Concerns with generative AI remain around — regulatory compliance, bias and ethical responses, data sharing, integration with legacy systems, talent shortage and high cost of training.
- These concerns can be addressed through 3 key factors:
- Culture – Embracing a cultural change, management support and investing in training and continuous learning.
- Accountability – Responsible by design, staying nimble and agile and incentivizing implementation.
- Maximizing the impact – Selecting the right use case, solving unique business problems for scalability and accepting failure.
- Due to regulatory scrutiny most use cases do not involve direct client-facing activities. This indicates the necessity of human oversight, despite efficiency and automation benefits.
- For firms embracing generative AI, or experimenting or still concerned with the technology, a good solution would be to partner.
- Partnerships provide access to specialized knowledge, tools, and best practices while tailoring adoption strategies, accelerating implementation, and mitigating common pitfalls.
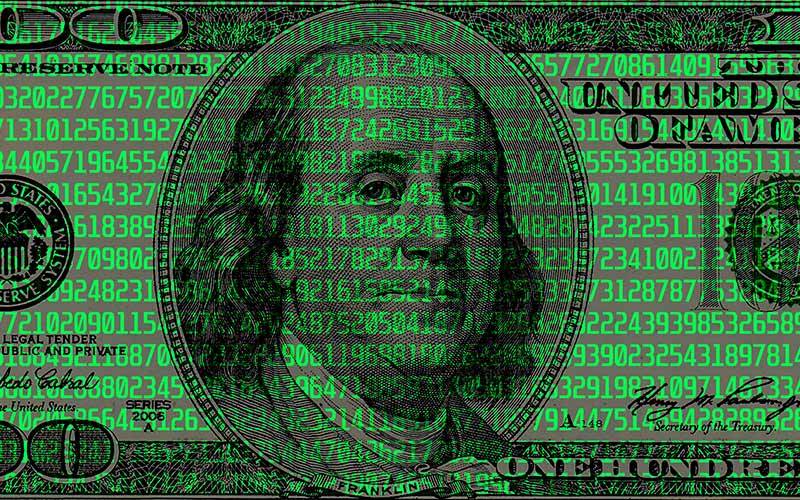
Value of generative AI
Generative AI is booming. ChatGPT reached a million users in just five days (Figure 1) and 100 million in 60 days – the fastest platform to do so. The market is currently worth around $14.2 billion and projected to soar to $111 billion by 2030. With applications around five foundational pillars — code, text, audio, video and images — generative AI is clearly a transformative technology.
Financial institutions are swiftly exploring generative AI, breaking free from their risk-averse reputation. Even firms with ingrained processes quickly and relatively easily incorporate generative AI into their workflow, often starting with pilot projects. Wide availability, easy deployment, and seamless integration with existing processes significantly improves productivity, explaining the popularity of generative AI. McKinsey sees generating value from increased productivity of 2.8% to 4.7% of the banking industry’s annual revenues, equivalent to an additional $200 billion to $340 billion.
Transformative potential in capital markets
The capital markets industry is ripe for change amid industry and technological headwinds. Customers, both existing and new, old and young, seek digital, convenient, and personalized experiences. The industry must transform to avoid falling behind. Nimbler, digital-native providers that cater to these preferences have risen, built on cloud with low legacy-tech baggage.
The industry has accumulated petabytes of both structured and nonstructured data that remain untapped. Margins are compressed due to a difficult global environment, advent of new players, and rising inflation. This has pushed costs to levels not seen in two decades, even as customers spend down their cash reserves. Between these challenges, incumbents face a rapidly changing regulatory landscape.
These factors prompt the industry to embrace generative AI. Top Wall Street firms are currently either piloting or actively deploying generative AI use cases. Deutsche Bank has 25 such use cases — from software development and managing adverse media to boosting junior banker productivity by automating tasks like analyzing comparables and company reports. McKinsey estimates that a diverse set of implementations could generate productivity gains of 30% to 90%, boosting operating profits by 9% to 15%. We believe these use cases broadly divide in four areas — building new capabilities, improving operations, fostering innovation, and enabling transformation.
By simulating different product scenarios and outcomes, generative AI assists firms to prototype and refine products. It processes vast datasets to pinpoint market trends and predict market movements, aiding in the development of investment strategies. JPMorgan’s ChatGPT-based model analyzes Federal Reserve speeches, potentially helping in policy change predictions and trading.
This tech enables personalized financial advice and recommendations to enhance customer experience. Morgan Stanley for example uses generative AI to help financial advisers answer investor queries. In another instance, Broadridge’s BondGPT streamlines the identification of corporate bonds on its LTX platform, increasing efficiency in bond selection and portfolio construction. This benefits asset managers, hedge funds, and dealers while also enhancing existing platforms.
Jim Crowley, global head of BNY Mellon Pershing, believes that generative AI has “enormous potential in wealth management.” BNY Mellon’s Wove equips wealth advisers with an interconnected experience that generative AI can amplify to build more actionable insights for clients.
Generative AI serves various other purposes, including fraud identification and secure software development. JPMorgan uses large language models (LLMs) on top of its fraud detection techniques, using pattern analysis to detect email compromise signs through contextual understanding. Goldman Sachs uses it to automate code testing and writing through English-language commands, improving productivity.
Market infrastructure players such as Nasdaq and London Stock Exchange Group too have begun to dabble with generative AI. The latter is working with Microsoft and several banks to create bespoke generative AI models in a move that shows how the financial services industry is looking to harness the technology without exposing proprietary data.
Generative AI is not without concerns
Generative AI has its limitations. The technology is fairly new with challenges to address before capital market firms adopt it. Major concerns are around data privacy, algorithmic biases, and job displacement. Additionally, the reliance on AI for decision-making raises questions about accountability and transparency in financial practices. Firms must ponder their investment returns at the same time as managing the transition from their complex legacy tech estates.
1. Is it reliable?
- Regulatory compliance: Capital markets are heavily regulated, and any generative AI product must also be compliant. Governance frameworks for AI use, including interpretability and accountability for AI-driven decisions, is necessary. While the regulatory environment for AI is still evolving, it creates uncertainty and compliance risks. However, regulatory bodies and governments have initiated measures in 2023 to address these concerns. In the US, the Securities and Exchange Commission (SEC) is increasingly scrutinizing AI-driven advice to ensure compliance and safeguarding investors’ interest. The US government issued an executive order to manage the risks associated with generative AI. For an industry generally considered to be hindered by regulations, this sector actively pursues measures to facilitate generative AI adoption. JPMorgan, for instance, is engaging with US regulators to explain their generative AI projects and ensure proper controls.
- Concerns around bias and ethical responses: LLMs “hallucinate” – create outputs that are not true – and learn and perpetuate biases into responses based on their training data, leading to unfair or discriminatory outcomes. Clearly defined ethical guidelines can address concerns around harmful content generation and ensure ethical AI usage, especially in sensitive areas like financial advice and risk assessment.
2. Is it tailored to capital markets?
- Data sharing in a regulated environment: Response quality of the technology correlates with input quality into the LLM. Most regulated capital market firms are reluctant to feed proprietary and client data into the LLM, thereby not allowing responses to be as precise as they can be. Morgan Stanley has “fine-tune” trained the GPT-4 model that underpins ChatGPT on thousands of company documents to build its own internal tool. This alleviates hallucination concerns and safeguards corporate IP.
- Integration with existing legacy systems: Capital market firms still operate on legacy systems and within data silos, causing potential integration issues with generative AI technologies. Upgrading or modifying these systems can be costly and time consuming.
3. Is it scalable?
- Talent shortage: Capital market firms have to compete with big tech for generative AI talent. There is a shortage of AI expertise that can build responsible, sustainable and productive solutions. An Infosys survey of North American businesses backs this up – lack of skills and talent is the biggest challenge for 24% of financial services firms. A key talent requirement here is prompt engineers, who straddle the boundary between programming and creative writing. They are very much in demand and highly compensated – Anthropic, Google’s latest $300 million investment, is hiring prompt engineers at salaries up to $335,000 a year, underscoring the value placed on prompt engineering and its role as a crucial skillset. Demand for roles such as generative AI applied scientist and multimodal generative modeling research engineer has risen, necessitating skills such as prompt engineering, AI language model training and engineering chains.
- High cost of training:Training and running an LLM is expensive, requiring huge amounts of energy: training GPT-4 is said to have cost more than $100 million. On top of that the “fine-tuning” process, which involves model adjustments, incurs a price based on the size of training, input, and output data. Prices are per 1,000 tokens or nearly 750 words. Additionally, maintaining an LLM can be especially burdensome for smaller firms. And demonstrating a return on investment on this spending in the short term can be challenging.
Adopt generative AI in capital markets
Despite the challenges, the potential advantages of generative AI make it difficult to ignore. For firms to adopt generative AI quickly yet securely, a blend of culture, accountability, and impact must be considered:
1. It is about culture
- Embrace cultural change: A culture of innovation is key to quick and successful adoption of generative AI. Traditional firms trade on their reputation for being safe, steady, and considerate — the opposite of what is needed to innovate. Adopting characteristics of testing quickly, failing fast, and changing with agility can give firms early breakthroughs. For instance, Morgan Stanley began its generative AI journey earlier than most firms.
A culture of innovation lays the foundation for becoming AI-first — understanding which experiences and processes to amplify through AI, the tooling and automation needed to deploy models and obtaining the right talent and operating model to bring this AI to life. - Management support: Executive buy-in and support are crucial to building a generative AI solution. They must also have appropriate oversight of AI systems with clear accountability with sufficient understanding of AI. An Infosys survey of businesses in the US and Canada found that one clear positive in this rapid embrace of generative AI is that it is being taken seriously by senior executives, and beyond the confines of the IT teams. Our survey reveals that both CEOs and leaders of business units play leading roles.
- Invest in training and continuous learning: A well-developed training program to equip staff with the necessary skills is foundational for large scale adoption. This could include internal training sessions, external certifications, and hands-on workshops like hackathons. Businesses should also foster a continuous learning environment to keep pace with evolving AI technologies and applications.
2. It is about accountability
- Responsible by design: Integrate ethical considerations into the design and architecture of generative AI solutions, particularly focusing on avoiding bias, protecting privacy, and preventing harmful content. Develop a responsible by design framework for ethical AI usage. Responsible by design AI is guided by principles such as human oversight and governance at every stage, constant auditing of processes and products for fairness and inclusiveness, and transparent, explainable, reliable, safe, secure, and private AI at each stage. Implement this through five building blocks: objective, governance, metrics, capabilities, and compliance.
- Stay nimble and agile: Design flexible and adaptable solutions to switch between different LLMs or technologies without significant overhauls. Keep abreast of generative AI developments to leverage emerging opportunities.
- Incentivize implementation: Implement incentive structures that encourage employees to engage with and leverage generative AI capabilities. Establish a rewards system for successful implementation and innovative use of the technology, promoting a culture of innovation and achievement.
3. It is about maximizing the impact
- Select the right use case: Select a use case that offers quick wins and addresses ongoing challenges without extensive fine tuning or prompt engineering. Opt for those with widespread application and clear, easily understandable success criteria.
- Solve unique business problems for scalability: After establishing the foundation of generative AI, solve unique industry-specific business problems. This can yield significant ROI in the short to medium term. This approach can also confer a competitive advantage as the technology matures.
- Accept failure: Cultivate a culture that tolerates and learns from failures, understanding that generative AI is rather new. Encourage rapid prototyping and iteration, allowing for quick learning and adjustment based on feedback and results.
Capital market firms cautiously adopt generative AI due to regulatory scrutiny around the industry. As a result, most use cases do not involve direct client-facing activities, such as delivering advice. This indicates the necessity of human oversight, despite efficiency and automation benefits.
While some firms wholeheartedly embrace generative AI across their business, including technology and operations, others are experimenting but hesitant to invest in a comprehensive strategy. A few remain concerned about technology reliability.
A solution for each of these categories of firms is to partner. Partnerships provide access to specialized knowledge, tools, and best practices while tailoring adoption strategies, accelerating implementation, and mitigating common pitfalls.